Generative AI seminar,
from 0 to up to 30% efficiency increase in business processes.
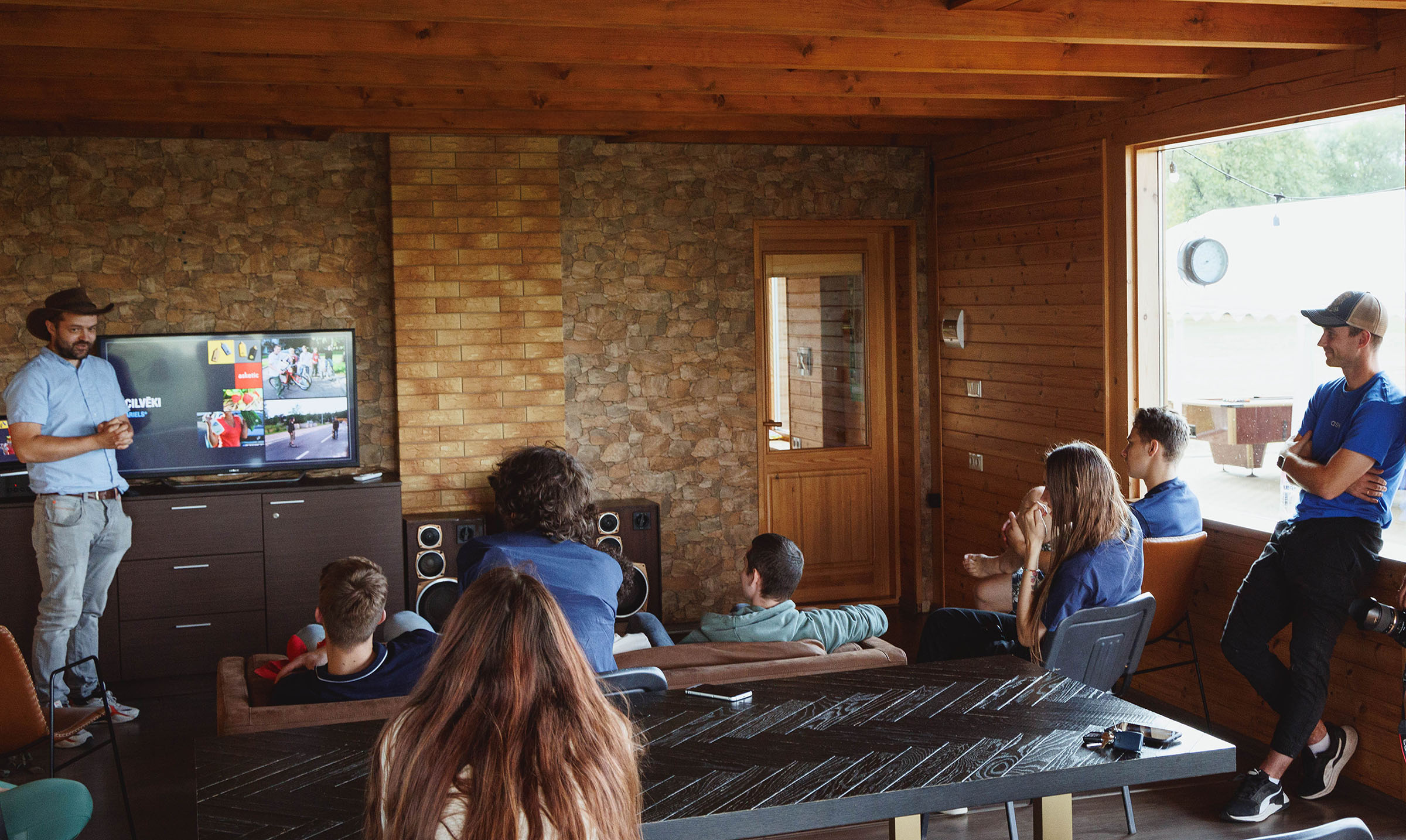
Seminar schedule
Learn how to integrate generative AI into your company or organization.
The seminar process begins with a simple setup: “What would I ask a new specialist who has to perform a specific task”. By getting acquainted with the fundamental nature of LLM functions, we will collectively understand what is possible, what the limitations are, and what is science fiction that sounds appealing to the ears but creates pitfalls for business.
We define an individual AI architecture and strategy.
When building a generative tools strategy, it's important to define the overall vision and be aware of the possibilities and limitations. For example, which LLM will we use? How much does it cost? What is available as an open-source solution? Where do we need unique models? How do we integrate them with our data sets? How do we improve learning and quality control in these models? How will we address data security issues?
A clear plan for applying generative tools to your needs.
To get ahead of the market, create your own unique AI solutions. It's no secret that successful companies have a significant amount of structured data. However, it often remains unused. This data is the perfect raw material for creating unique AI tools.
Experience
By learning how generative AI technology works (compared to traditional IT solutions), we discovered a new way to improve the usability of our digital product. As a result, we achieved about 30 hours of savings on customer training. And 25% greater subsequent engagement from the customer. Also, a new method for monitoring system usage.
Edgars Lacis, Sales Director of Pitch Patterns
Plan the Eldigen Generative Tools Seminar.
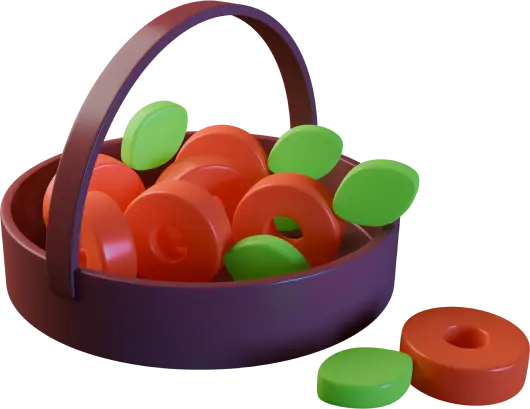
Activating LLM generative models occurs within a secure enterprise-focused process.
Activating LLM for enterprises begins with model selection tailored to specific industries, followed by integration into the company's IT systems. Security is a key aspect of this process, including data encryption and access control, to protect both input data and generated results. The process concludes with regular model performance and security checks to ensure it meets the company's requirements and regulatory standards, while maintaining data security and confidentiality.
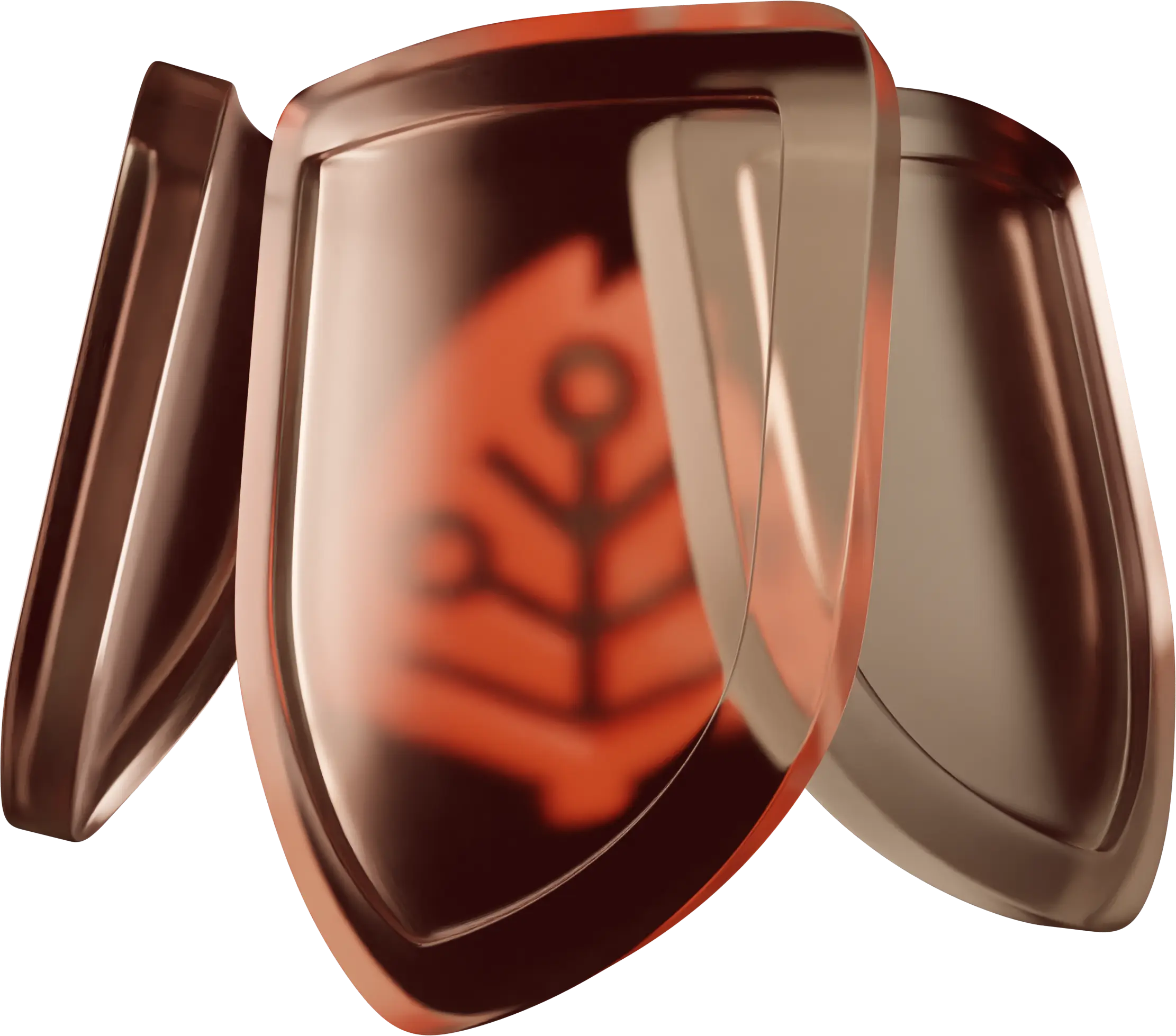